The Allen Institute for AI (Ai2) released a new tool that links AI-generated text to training data, aiming to improve transparency and accountability in artificial intelligence by addressing one of the biggest mysteries in the field.
Ai2 says the project, OLMoTrace, goes further than any other tool to show how the data used to train large language models may influence what they generate.
It converts “a black box to a glass box,” said Ali Farhadi, CEO of the Seattle-based nonprofit, during a briefing with reporters.
OLMo Trace highlights phrases from the AI’s responses that appear verbatim in the training data, and links users to the original sources. The idea is to support basic fact-checking and a better understanding of how the model was influenced by specific documents.
The tool, announced Wednesday in conjunction with the Google Next cloud conference, builds on Ai2’s open approach to AI development, capitalizing on its practice of publicly releasing training data, code, model weights, and other components of AI models. This level of traceability isn’t possible with most commercial systems.
Ai2 is making OLMoTrace itself open-source so anyone can use it, improve it, or build on it. The larger goal is to improve understanding of how AI models work and make them easier to trust.
The question of exactly how AI models generate their responses has loomed over AI since the original release of OpenAI’s ChatGPT in November 2022.
So what has Ai2 learned from peering into the black box?
While they’ve yet to reach sweeping conclusions, researchers so far have seen examples of AI models repeating patterns the models have seen in training, rather than coming up with answers through reasoning. In some cases, what looks like problem-solving is really just the model copying an example it was trained on.
For example, Ai2 researchers looked into a math problem that Anthropic had analyzed in a recent blog post: 36+59. While Anthropic traced the answer to complex internal processes inside the model, Ai2 found that the problem and answer appeared multiple times in its training data.
Since many AI models are trained on similar datasets, this means it’s possible that the model didn’t solve the problem on its own — it simply repeated an answer it had seen before.
In other words, it’s the AI equivalent of looking over someone else’s shoulder during the quiz.
In some ways, revealing how the “trick” works might make AI seem less mysterious or magical, Ai2 researchers acknowledged. But they said it’s also impressive to see how much information AI models can retain from training, and how much knowledge is embedded in their weights.
Can this approach explain AI hallucinations? Yes, at least in some cases. Ai2 researchers are finding that AI mistakes or false claims can sometimes be traced back to bad or misleading information in the training data.
“Some documents just contain wrong things, non-factual things, that went into the training pipeline of the model and ended up misleading the model,” said Jiacheng Liu, the lead researcher behind OLMoTrace at Ai2, and a Ph.D student at the University of Washington.
Ai2 researchers say OLMoTrace does not prove a direct causal link between a document and a model’s output. Instead, it suggests that the model probably drew from that language during training.
“Scientifically, we won’t say this is the cause, but intuitively, it makes sense. When it has been seen in the training data, it makes sense,” said Hanna Hajishirzi, senior director of NLP research at Ai2, and a UW Allen School associate professor of computer science.
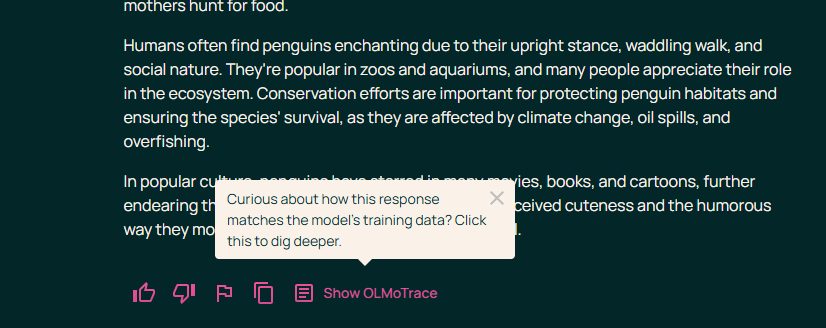
To access OLMoTrace, users can generate a response from one of Ai2’s open-source language models in the Ai2 Playground. A “Show OLMoTrace” button appears below the output. When clicked, the tool highlights any phrases that appear verbatim in the model’s training data. A panel to the right links to the original documents where those phrases were found.
Ai2 researchers say OLMoTrace could be helpful in areas like healthcare, finance, and science, where people and companies need to know where information comes from and be able to check it. In some cases, the tool could also make it easier to meet rules and regulations by showing what data may have shaped an AI’s answer.
Ai2 says OLMoTrace is different in its approach from other tools that link to source materials. Perplexity.ai, for example, finds documents first and uses them to help the AI write its answer.
OLMoTrace takes the opposite approach — it reviews the model’s answer after it’s generated, then checks to see if any parts came directly from the training data. The goal isn’t to guide the model, but to help people understand where its answers might be coming from.
Ai2, founded in 2014 by the late Microsoft co-founder Paul Allen, is funded by the Allen estate and other donors. The nonprofit developed and released 111 artificial intelligence models in 2024, and announced its participation in the AI Cancer Alliance last week.
Read the full article here